New algorithms for data privacy achieve higher utility than current methods and withstand attacks by machine learning
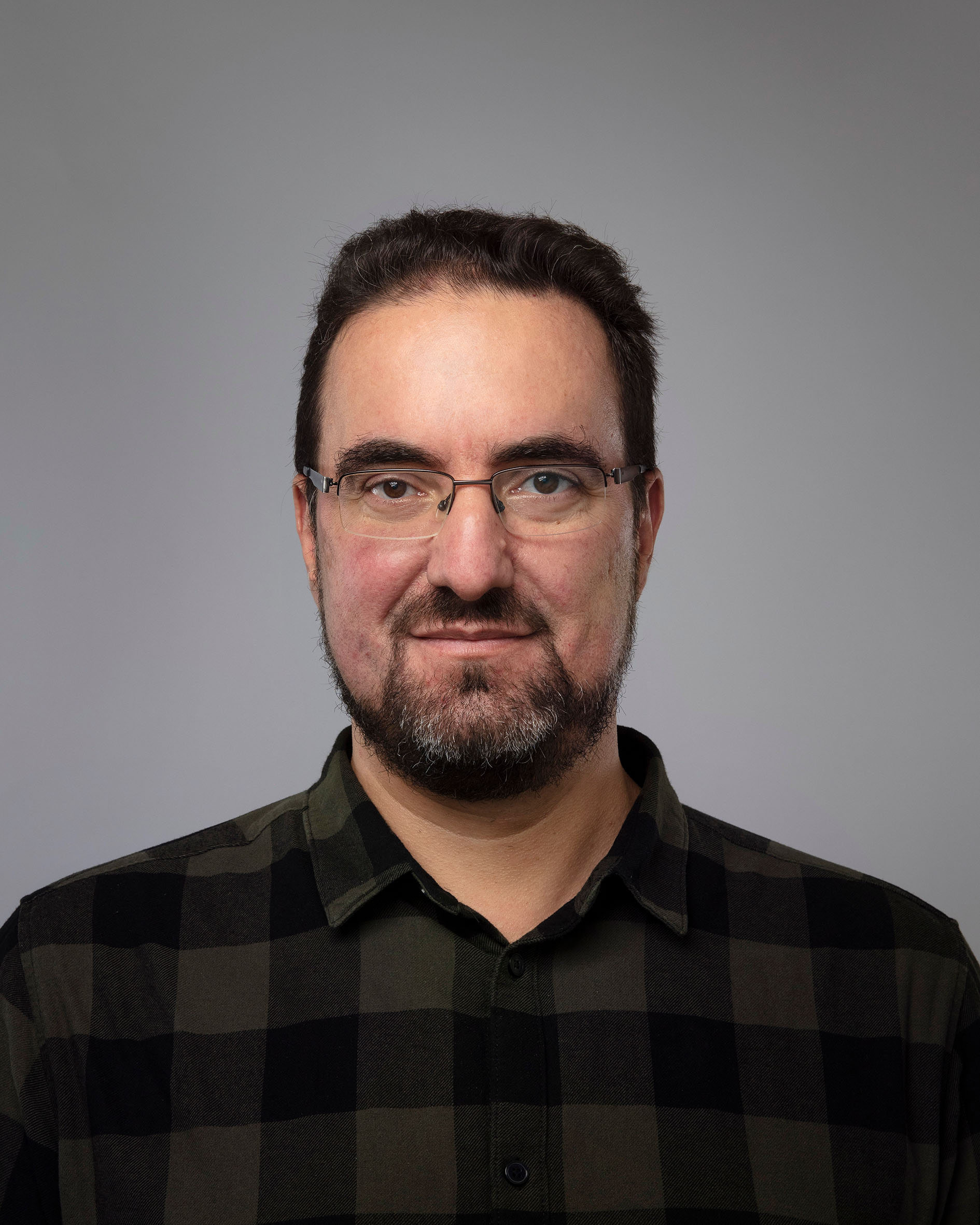
A new paper by associate professor Panagiotis Karras, and co-authors from University of Liverpool and National Technical University of Athens and PhD graduates of the National University of Singapore, revives the interest in an alternative view to privacy. In short, instead of bounding a general privacy risk for each individual who shares her data separately (as differential privacy does), it bounds a specific privacy risk for all individuals at the same time. The paper was recently published at the USENIX Security conference.
How can we orchestrate an one-off sharing of informative data about individuals, while bounding the risk of disclosing sensitive information to an adversary who has access to the global distribution of such information and to personal identifiers? Despite intensive efforts, current privacy protection techniques fall short of this objective. In their paper Karras et al. develop algorithms for disclosure control that protect sensitive information and, at the same time, gain up to 77% in data utility against current methods using a bipartite matching blueprint to blur the exact correspondence between data values and individuals. Moreover, this method obstructs attempts to infer the specific protected values by machine learning.
Read the full paper at: https://www.usenix.org/conference/usenixsecurity22/presentation/gkountouna