Hans-Jörg Schulz Secures Grant for Innovative EEG Data Project
In a significant development for the field of computer-supported visual artifact detection in EEG data, Associate Professor Hans-Jörg Schulz has been awarded a grant of DKK 2,878,568 from Independent Research Fund Denmark (Danmarks Frie Forskningsfond). The grant will fund the project "ArtiPlex: Multiplex Analytics for Computer-supported Visual Artifact Detection in EEG Data."
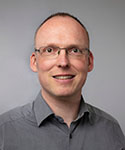
The primary challenge in EEG (Electroencephalography) data analysis lies in identifying and mitigating artifacts—distortions in the signals that span spatial, frequency, and temporal scales. Defining what constitutes an artifact and determining the threshold for normal data is inherently complex and often depends on the method of data collection. As a result, semi-automated, human-in-the-loop approaches are commonly employed. These methods pre-screen EEG data automatically for artifacts, and domain experts subsequently correct the output. While effective, this process is time-consuming and labor-intensive.
The ArtiPlex project seeks to revolutionize the current artifact detection paradigm by integrating the results of multiple detection methods running in parallel. The key innovation lies in proposing Multiplex Analytics—a concept designed to progressively visualize the stream of automated detection results. This approach allows experts to monitor and steer automated processes effectively.
Through a collaborative effort between Associate professor Hans-Jörg Schulz from Department of Computer Science and Assistant professor Kaare Mikkelsen from Department of Electrical and Computer Engineering at Aarhus University, ArtiPlex aims to explore visually interactive methods to enhance artifact detection. The project goes beyond displaying the combined result of a single automated detection method, instead showcasing the outcomes of various methods. This multiplex approach promises to ease artifact detection in EEG data, leading to faster semi-automated preprocessing of this vital information.