ALCOMFT-TR-02-84
|
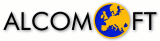
|
José L. Balcázar, Yang Dai and Osamu Watanabe
Provably fast support vector regression using random sampling
Barcelona.
Work package 1.
May 2002.
Abstract: Support Vector Machines are a family of data analysis algorithms,
based on convex Quadratic Programming. Their use has been demonstrated
in classification, regression, and clustering problems. In previous
work we have proved that a random sampling technique based on an
evolving discrete probability distribution provides a training
algorithm for Support Vector Classification with provably low
expected running time as a function of the number of points.
This paper proves that the same technique can be applied to
Support Vector Regression, by applying and reinterpreting an
intuitive geometric breakthrough by Bi and Bennett, and obtaining,
through the use of such discrete techniques, the first (to our
knowledge) support vector regression algorithm with a provably
low expected running time as a function of the number of examples.
This is expected to be useful for the processing of extremely large
datasets.
Postscript file: ALCOMFT-TR-02-84.ps.gz (95 kb).
System maintainer Gerth Stølting Brodal <gerth@cs.au.dk>